Most Financial Research Is Probably Wrong, Say Financial Researchers
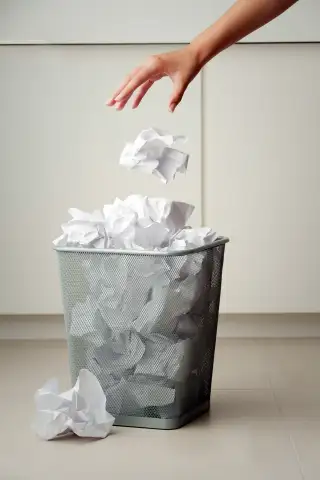
In the 1990s, when I first stated writing about investing, the stars of the show on Wall Street were mutual fund managers. Now more investors know fund managers add costs without consistently beating the market. So humans picking stocks by hand are out, and quantitative systems are in.
The hot new mutual funds and exchange-traded funds are scientific—or at least, science-y. Sales materials come with dense footnotes, reference mysterious four- and five-factor models and Greek-letter statistical measures like "beta," and name-drop professors at Yale, MIT and Chicago. The funds are often built on academic research showing that if you consistently favor a particular kind of stock—say, small companies, or less volatile ones—you can expect better long-run performance.
As I wrote earlier this year, some academic quants even think they've found stock-return patterns that can help explain why Warren Buffett has done so spectacularly well.
But there's also new research that bluntly argues that most such studies are probably wrong. If you invest in anything other than a plain-vanilla index fund, this should rattle you a bit.
Financial economists Campbell Harvey, Yan Liu, and Heqing Zhu, in a working paper posted this week by the National Bureau of Economic Research, count up the economic studies claiming to have discovered a clue that could have helped predict the asset returns. Given how hard it is supposed to be to get an edge on the market, the sheer number is astounding: The economists list over 300 discoveries, over 200 of which came out in the past decade alone. And this is an incomplete list, focused on publications appearing in top journals or written by respected academics. Harvey, Liu, and Zhu weren't going after a bunch of junk studies.
So how can they say so many of these findings are likely to be false?
To be clear, the paper doesn't go through 300 articles and find mistakes. Instead, it argues that, statistically speaking, the high number of studies is itself a good reason to be more suspicious of any one them. This is a little mind-bending—more research is good, right?—but it helps to start with a simple fact: There's always some randomness in the world. Whether you are running a scientific lab study or looking at reams of data about past market returns, some of the correlations and patterns you'll see are just going to be the result of luck, not a real effect. Here's a very simple example of a spurious pattern from my Buffett story: You could have beaten the market since 1993 just by buying stocks with tickers beginning with the letters W, A, R, R, E, and N.
Researchers try to clean this up by setting a high bar for the statistical significance of their findings. So, for example, they may decide only to accept as true a result that's so strong there's only a 5% or smaller chance it could happen randomly.
As Harvey and Liu explain in another paper (and one that's easier for a layperson to follow), that's fine if you are just asking one question about one set of data. But if you keep going back again and again with new tests, you increase your chances of turning up a random result. So maybe first you look to see if stocks of a given size outperform, then at stocks with a certain price relative to earnings, or price to asset value, or price compared to the previous month's price... and so on, and so on. The more you look, the more likely you are to find something, whether or not there's anything there.
There are huge financial and career incentives to find an edge in the stock market, and cheap computing and bigger databases have made it easy to go hunting, so people are running a lot of tests now. Given that, Harvery, Liu, and Zhu argue we have to set a higher statistical bar to believe that a pattern that pops up in stock returns is evidence of something real. Do that, and the evidence for some popular research-based strategies—including investing in small-cap stocks—doesn't look as strong anymore. Some others, like one form of value investing, still pass the stricter standard. But the problem is likely worse than it looks. The long list of experiments the economists are looking at here is just what's seen the light of day. Who knows how many tests were done that didn't get published, because they didn't show interesting results?
These "multiple-testing" and "publication-bias" problems aren't just in finance. They're worrying people who look at medical research. And those TED-talk-ready psychology studies. And the way government and businesses are trying to harness insights from "Big Data."
If you're an investor, the first takeaway is obviously to be more skeptical of fund companies bearing academic studies. But it also bolsters the case against the old-fashioned, non-quant fund managers. Think of each person running a mutual fund as performing a test of one rough hypothesis about how to predict stock returns. Now consider that there are about 10,000 mutual funds. Given those numbers, write Campbell and Liu, "if managers were randomly choosing strategies, you would expect at least 300 of them to have five consecutive years of outperformance." So even when you see a fund manager with an impressively consistent record, you may be seeing luck, not skill or insight.
And if you buy funds that have already had lucky strategies, you'll likely find that you got in just in time for luck to run out.